
Above: “data privacy” by stockcatalog licensed under CC BY-SA 2.0
TokenRank: a rating method for digital art
Foreward (by Chiara Braidotti)
Throughout history, art and value have always been intertwined concepts, and many actors within and beyond the art world have studied the dynamics underpinning this association. But is there an objective, standardized means to determine this relationship and, perhaps, inform it? In other words, can we actually measure the value of art?
Of course, there is no univocal answer to such a question. Some might say this problem simply cannot be solved, as it involves too many variables; others might argue it should not be solved, for to reveal the secret behind the alchemy at issue might deprive art of its mystery. Actually, this question would demand us to discuss the very definition of both art and value themselves in the first place, if we had the ambition to address it properly.
In any case, however we look at the matter, it will appear that knowledge and research are crucial to our understanding of arts and culture and their value. When considering the crypto art genre, whether we wish to determine the relevance and meaning of a piece or its monetary price, the influence of background data and mined information on these aspects is undeniable.
The rating problem
In material or traditional art, each artist has a rating that is earned over the years and that depends on several factors, including: auction bids and sales, presence of the artworks in national and international galleries, public and museum acquisitions, awards received, publications in art magazines, reviews and impressions of art critics, exhibitions in galleries that have hosted the artist, solo and group exhibitions. The rating of an artwork is directly determined by the rating of the artist that created it, as well as by the size and the materials of the art piece itself. Nevertheless, there is no accepted standard for the compilation of these ratings; moreover, often this information is secreted or available only for a (significant) fee.
Blockchain has been used to deal with a central challenge to selling digital art: how to create a limited edition of a file that can be easily reproduced. Crypto art is a rising art movement in this cypher space. It associates digital artworks with unique and provably rare tokens that exist on the blockchain; these codes are the equivalent of the artist’s signature. The real potential of the emerging crypto art current is to give a digital image the dignity of a true work of art, made unique, eternal and collectible through blockchain technology.
At the moment there is neither a standard nor any shared proposal for defining ratings for crypto artists and artworks. This neatly contrasts with the public availability of the entire data flow of crypto art, including artwork images, artwork metadata and trade transactions (bids and sales). All this data is accessible on the Ethereum blockchain. For free.
A bag of artworks
We assume a general setting in which artworks are created by artists and displayed in a digital gallery. Artists can set a price for the displayed artworks. Collectors can directly buy the artwork at the set price or make an offer (a bid) for an artwork. Artists can accept the highest bid for a listed artwork. When sold, the artwork remains tradable on the secondary market of the gallery.
In our view, the first-class citizen in art is the artwork, the creativity product of artists that collectors long avidly. Hence, we will mainly focus on artworks, and indirectly on artists and collectors:
- we define a statistically sound method to rate an artwork on a digital gallery;
- for the sake of the assessment, we view artworks and collectors as bags of artworks: the artist is associated with the set of created artworks, the collector with the set of collected. artworks.
The method can be applied to other entities besides artists and collectors, for instance:
- the bag of all artworks created by artists in a digital gallery within a given time frame, for instance in a month. This opens the possibility of assessing the gallery collection dynamically over time;
- a random bag of artworks in a gallery. We can use this as a reference benchmark for evaluation.
A rating method for artworks
Hence, there are two major signals of market success for an artwork:
- the bid history, composed of all priced bids and bidders
- the sale history, made by all priced sales on primary and secondary market
It is worth stressing that one important facet of an artwork is the artist that created it. One might consider assessing each artist using the very same rating of the artworks he or she created and then feed the rating method for an artwork with this additional parameter. However, this creates a feedback loop difficult to solve: the artwork score depends on its creator’s score, which in turn depends on the scores of the artworks he or she created. In computer science, we say that in this case artworks and artists are mutually recursive. Although there are mutual recursive systems that have a sound solution, for instance Kleinberg’s HITS to rate Web pages, the mathematical specification of the artwork-artist problem is, at the moment, not clear to us (suggestions are welcome!). On the other hand, one might use a different method to rate artists, not related to the rating method for artworks. We made a proposal in this paper, which is currently under review at Communications of the ACM.
The inclusion of an artist’s score in the rating for an artwork has, however, pros and cons. For instance, suppose a foresighted collector scouts and acquires for a few cents the artworks of an emerging artist who eventually becomes a blue chip. If the collector has strong hands and holds the collected artworks, the sale (and possibly bid) history of the collected artworks is limited, and hence these artworks do not increase their value in terms of metrics. This issue is mitigated if we include the rating of the artist in the assessment of the artwork. Indeed, as the artist climbs the ranks, their artworks do too. On the other hand, notice that both the bid and sale history already contain, indirectly, the artist facet. An artwork created by a blue-chip artist will reasonably receive more and higher bids and will be sold for higher prices and maybe more times. Hence, including the artist in the assessment of the artwork has the risk of overloading the artist factor and reducing the game competition to the usual suspects (we all know). And in fact, our experiments confirm this bias.
An important caveat is how to assess the actual price of sales and bids. Since digital artworks are mainly traded in crypto currencies, and these coins are not stable (they show large variance of the historical prices), we decided to use the price expressed in fiat money (dollars) at the exchange rate of the time of the bid or sale.
Specifically, we used the following metrics to assess the bid and sale history of an artwork:
- α: the sum of largest bids received by the artwork from each bidder
- β: the total number of bids received by the artwork
- γ: the number of different bidders that bid for the artwork
- δ: the sum of the sell prices of all sales (on primary and secondary market) made by the artwork
For example, suppose the bid and sale history of an artwork is as follows:
- bid of 1 from A
- bid of 2 from B
- bid of 3 from A
- bid of 4 from B
- bid of 5 from C
- sale for 5 to C
- bid of 6 from A
- sale for 6 to A
Here, collector A is willing to pay 6 (their largest bid) for the artwork, collector B would pay 4, and collector C would spend 5. Hence, the sum of largest bids on the artwork is α=6+4+5=15α=6+4+5=15 and represents a sort of open interest for the piece. The total number of bids is β=6β=6 and the number of different bidders is γ=3γ=3 (A, B and C). The sum of all sales is δ=5+6=11δ=5+6=11.
We thus define a global rating ρ for an artwork t as a weighted average of the above four metrics:
This opens an additional issue. In order to obtain a meaningful mean (we don’t want to sum apples with pears), we need to normalize these metrics first. Normalization is tricky since we noticed that, as expected, all these metrics have a long-tail distribution: the majority of artworks received low values for them while a significant share of blue-chip artworks have very large values. A long-tail distribution is skewed and differs from the well-known symmetrical normal distribution. For long-tail distributions, the mean is not representing the typical value, and hence we cannot use this approach for normalization. Hence, we used a percentile approach instead. Essentially, we assess each metric with the actual percentile (a number between 0 and 1) of the value of the metric for an artwork with respect to the entire distribution of the metric values for all artworks. For instance, if one artwork received 0 bids, and hence it scores 0 for all the bid metrics, but it is in good company, for instance a share of 25% of artworks are in the very same situation, then its percentile is 0.25 for all bid metrics (and not 0 as it would be using an average approach).

AI Generated Nude Portrait #1 by Robbie Barrat is the artwork with the highest global rating in the gallery (at the moment).
Given a collection of artworks SS, we further define the cumulative rating σσ of SS as
and the average rating μμ of SS as:
where |S| is the number of elements of S. Notice that σ depends on the size of the collection S while μ does not.
In the following, we describe three use cases of the proposed rating method on the dataset of all artworks from digital gallery SuperRare (two years of activity, from April 2018 to May 2020).
Use case I: rate a collection of artworks
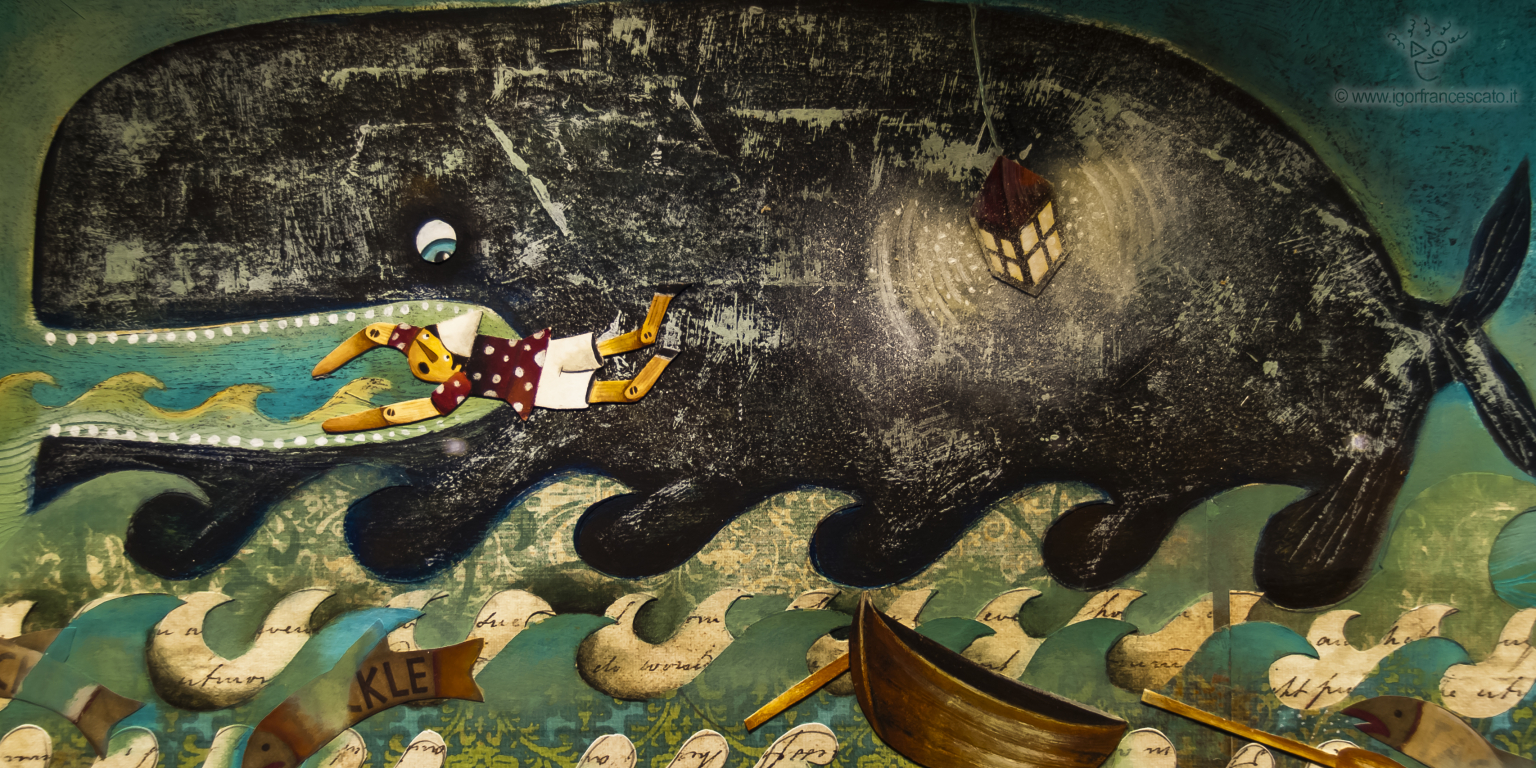
Pinocchio and the whale (image courtesy of Igor Francescato)
Recently, the major non-fungible token (NFT) collector WhaleShark launched a social currency with a foundation valuation that is underpinned by his large collection of NFTs. One of the issues related to this ambitious project is to make a fair, objective appraisal of the assets contained in the collection. WhaleShark declared he spent 5,000 ETH to make the collection.
Here, we use the above described assessment method on the assets of the collection that have been bought on SuperRare (this is only a fragment of the whole collection which also includes virtual land and games, as well as artworks purchased on different crypto art marketplaces). The SuperRare collection of WhaleShark is called, symptomatically, The Vault and contains, at the writing time, 443 digital artworks.
We compared the WhaleShark collection with another notable collection in the gallery (moderats’ collection). TheVault is neatly superior to moderats’ collection in terms of cumulative rating (σ=349σ=349 for TheVault and σ=221σ=221 for moderats) but it is comparable in terms of average rating (μ=0.789μ=0.789 for TheVault and μ=0.758μ=0.758 for moderats). We also matched the WhaleShark collection with a random collection of the same size. A random collection is a kind of benchmark and approximates the rating of the entire gallery as a collection. However, since it has the same size of the original collection, it is comparable with TheVault. In fact, we generated not just one but 1000 random collections and took the average of the ratings among random samples. In turns out that TheVault scores 1.34 times above the random benchmark.
Use case II: rate artists by artworks they created
In this second use case we rate an artist by assessing the value of the works they created. This is not as trivial as it seems, for we cannot simply assess the set of all works created by each artist, since: 1. artists create at different rates: there are artists that tokenize a new piece each day of the week and other that mint once a month; 2. artists have different histories: some have long been active in the space while others just landed there.
It is known that it is not statistically sound to compare means over samples of sizes that differ largely. Hence, we adopted a top-n-min-k approach. Given numbers kk and nn with k≥n≥1k≥n≥1:
- we select only artists that created at least kk pieces;
- for them we select the best nn artworks according to the rating ρρ;
- finally, we rate an artist using the mean rating μμ of the selected artworks.
A high value for nn favors artists with a long activity history; on the other hand, a small value for nn is inclusive with respect to artists with a short activity history, including emerging ones. We computed 3 rankings:
- blue-chip artists: k=n=30;
- green-chip artists: k=n=15;
- red-chip artists: k=n=5.
Use case III: rate the temporal evolution of a gallery
How does the rating of SuperRare artworks evolve in time? Does COVID-19 have an influence on this rating (bid and sale history)? We divide the last two years of SuperRare activity into 25 temporal slices of 30 days each. For each slice, we retrieve the artworks tokenized in that period and then compute the cumulative rating (σ) of the set. Notice the effect of the arrival of whales (big collectors) during last fall and that of COVID-19.

Coda
Besides market, there are other facets to appraise an artwork, including the text (title, description and keywords) accompanying the artwork as well as the features of the digital object itself (image, gif, video, sound). Furthermore, the judgement of art experts is another important variable that one can through on the table.
Articles Can Machine Learning Predict the Price of Art at Auction?, by Jason Bailey, and Machine Learning, Human Experts, and the Valuation of Real Assets by Mathieu Aubry et al. explore deeper this issue. We are working towards an integration of these features (text, image and curatorial assessment) into an inter-gallery rating system.
Art

Curated Conversations: ALIENQUEEN
SuperRare Labs Senior Curator An interviews ALIENQUEEN about psychedelics, death, and her journey in the NFT space.
Tech


Out of the Vault and onto the Chain: the Evolving Nature of Provenance
SuperRare editor Oli Scialdone considers the social experience of provenance and its relationship with community in the Web3 space.
Curators' Choice



Curated Conversations: ALIENQUEEN
SuperRare Labs Senior Curator An interviews ALIENQUEEN about psychedelics, death, and her journey in the NFT space.